Data Services
Make the most of your data. Power growth, innovation, and competitive differentiation.
Todays top performing businesses have put data at the foundation of their business model, knowing well that data helps solve tough problems, enables their leaders to make better decisions with greater confidence, and drive innovation that propels the organization to industry leadership.
Our Data Services are tailored to businesses at all stages of data adoption, with a common goal to accelerate an organization's data strategy through the addition of thought leadership, infrastructure foundations and automation, and identification and realization of the data opportunities unique to a business.
Data Strategy
Identify how your organization can capitalize on the big data movement. Develop a strategy tailored to what is unique about your data and business.
Data Analytics
Turn data into actionable insights with a comprehensive and serverless data analytics platform.
Exploratory Data Analysis
With an Exploratory Data Analysis, you can turn an almost unusable dataset into a completely usable dataset, and in the process, identify the relationships in the data that can power Machine Learning innovations.
Data Integration
Are your datasets in siloes or difficult to access? Could you benefit from integrating additional data? Connect multiple data sources and automate stream or batch ingestion on a schedule or in real-time. Hands-free, stress-free data integrations.
Data Platforms
Consolidate data in various formats, stored in various locations, with varying update frequencies to democratize access and produce the volume and quality of datasets required for machine learning.
Stream and Batch Systems
Make data more organized, useful, and accessible from the instant it is generated. Stream in real-time or batch upload from source to destination. Perform ELT or ETL. Achieve unlimited scale with Google Cloud Dataflow.
Data Warehouses
Consolidate and unify your diverse data sets in a central storage location. Democratize insights by enabling easier data access and retrieval with a secure and scalable platform.
Data Visualization
Analyze and understand complex data sets through the use of visual representations. Identify patterns, trends, and relationships within data. Gain valuable insights to inform decision-making and strategy development.
Machine Learning Operations
Cloud architectures to support the needs of production ML including model development, data processing, automated training, model deployment , workflow orchestration, artifact management, and model monitoring.
Cloud Infrastructure Services
Accelerate your business with digital technology. Adopt faster, more efficient, and more flexible infrastructure.
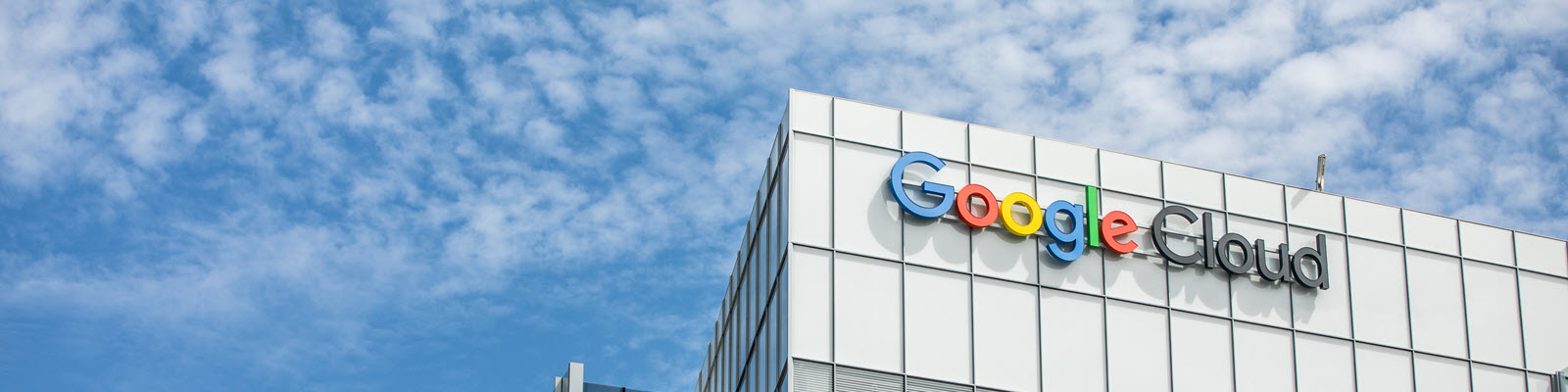
Businesses who welcome tech-driven change are better off, statistically, in wide range of areas including financials, operations, people, and culture. Cloud infrastructure introduces the ability for your business to develop new digital business lines, realize operational efficiencies, improve performance, and attract elite talent.
Our Cloud Infrastructure Services are designed to meet you where you are. On premise? Legacy Systems? In the cloud, but not happy or not optimized? Our team of certified Professional Cloud Architects will help you architect and implement an enterprise grade cloud environment, with an emphasis on security, governance, and scalability. Let us help you migrate, retool, improve, or advance your cloud.
Event Driven Infrastructure
Design and develop cloud-based, event-driven architectures. Make use of production grade functionality including Delivery Guarantees, Order/Duplicate handling, Filtering/Fanout techniques, Stream History and Replays, and more.
Site Reliability
Complete a migration or greenfield development with our Site Reliability advisory alongside. Adopt best practices in DevOps, security, networking, and performance optimization to meet SLAs.
Kubernetes Engine + Anthos Service Mesh
Build a scalable, distributed system using the right tools. Pair Anthos Service mesh with GKE to simplify service delivery, from traffic management and mesh telemetry to securing communications between services.
Development & Managed Services
Services to develop new products or manage critical operations
Engage our team to develop a new application, evolve an existing application, or manage and maintain your application or cloud environment.
We bring you the peace of mind and confidence, that your vision for your product is realized, or your existing product/environment is well maintained, performant, and continues to operate with success.
Application Development
A DataForce is a specialized MLOps team hired to lead your data and ML initiatives. Teams are staffed with proven expert leaders and engineers. Service includes execution and transformation. 50% savings vs in-house hire. Paid monthly.
Hypercare
Certified Google Professional Cloud Architects are the most scarce and expensive IT role. Gain unlimited, on-demand access to our growing "bench" of GCP architects. Annual cost is less than (1) in-house hire. Paid monthly.